Case Study
Behaviour Interactive
Leveling the playing field
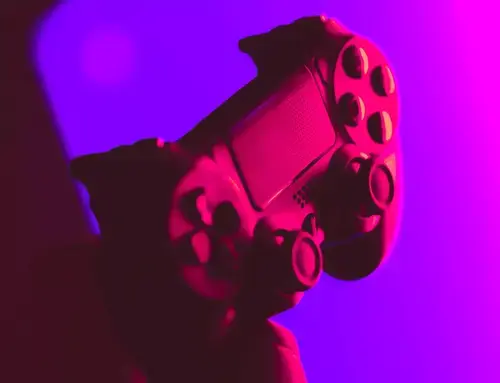
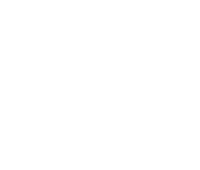
Helping a top gaming studio build more engaging games with the right data
Celebrating its 30th year, Behaviour Interactive has enjoyed immense success with its original IP Dead by Daylight, which now has more than 50 million players around the world and across all platforms.
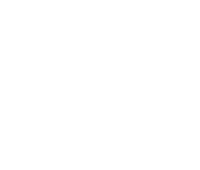
Helping a top gaming studio build more engaging games with the right data
Celebrating its 30th year, Behaviour Interactive has enjoyed immense success with its original IP Dead by Daylight, which now has more than 50 million players around the world and across all platforms.
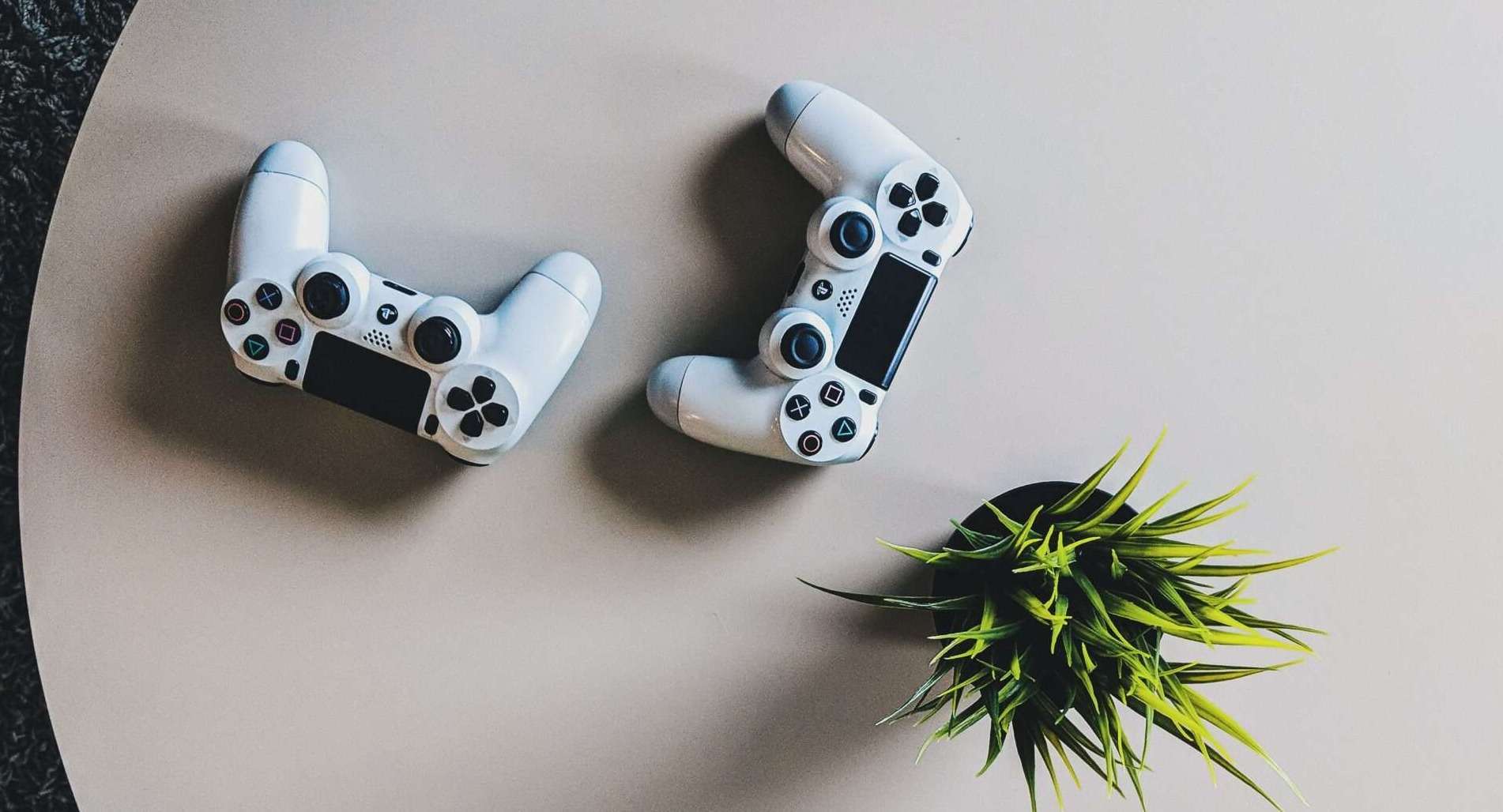
The team at Behaviour leverages game data to determine which features should be developed to create more engaging experiences so as to optimize the profitability of their games.
To achieve this outcome, Behaviour needs to develop a keen understanding of player behavior such as:
- When they play
- How long they play for
- Why they ended their session
- Which character they select
- What options they prefer
- How they progress through the game
Additionally, understanding the impact of monetization at various stages of the game is crucially important; players can opt to pay real money to unlock in-game valuables such as weapons and costume upgrades. Understanding what the player buys, when they buy it and why they buy it is highly valuable knowledge.
Ultimately, the end goal of the Behaviour Interactive team is to build more engaging and profitable games.
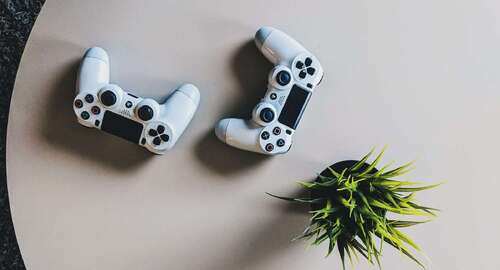
The team at Behaviour leverages game data to determine which features should be developed to create more engaging experiences so as to optimize the profitability of their games.
To achieve this outcome, Behaviour needs to develop a keen understanding of player behavior such as:
- When they play
- How long they play for
- Why they ended their session
- Which character they select
- What options they prefer
- How they progress through the game
Additionally, understanding the impact of monetization at various stages of the game is crucially important; players can opt to pay real money to unlock in-game valuables such as weapons and costume upgrades. Understanding what the player buys, when they buy it and why they buy it is highly valuable knowledge.
Ultimately, the end goal of the Behaviour Interactive team is to build more engaging and profitable games.
The challenge
Behaviour operates a 150TB data lake that sees approximately 300GB of data movement on any specific day.
Their existing data pipeline was a custom solution built on top of Amazon Web Services (AWS) services, ingesting files through Kinesis Firehose, storing them in S3 and making them available for querying via Athena. While this solution had its advantages, it was difficult to maintain and lacked some core functionalities to meet the emerging requirements of the Player Insights team.
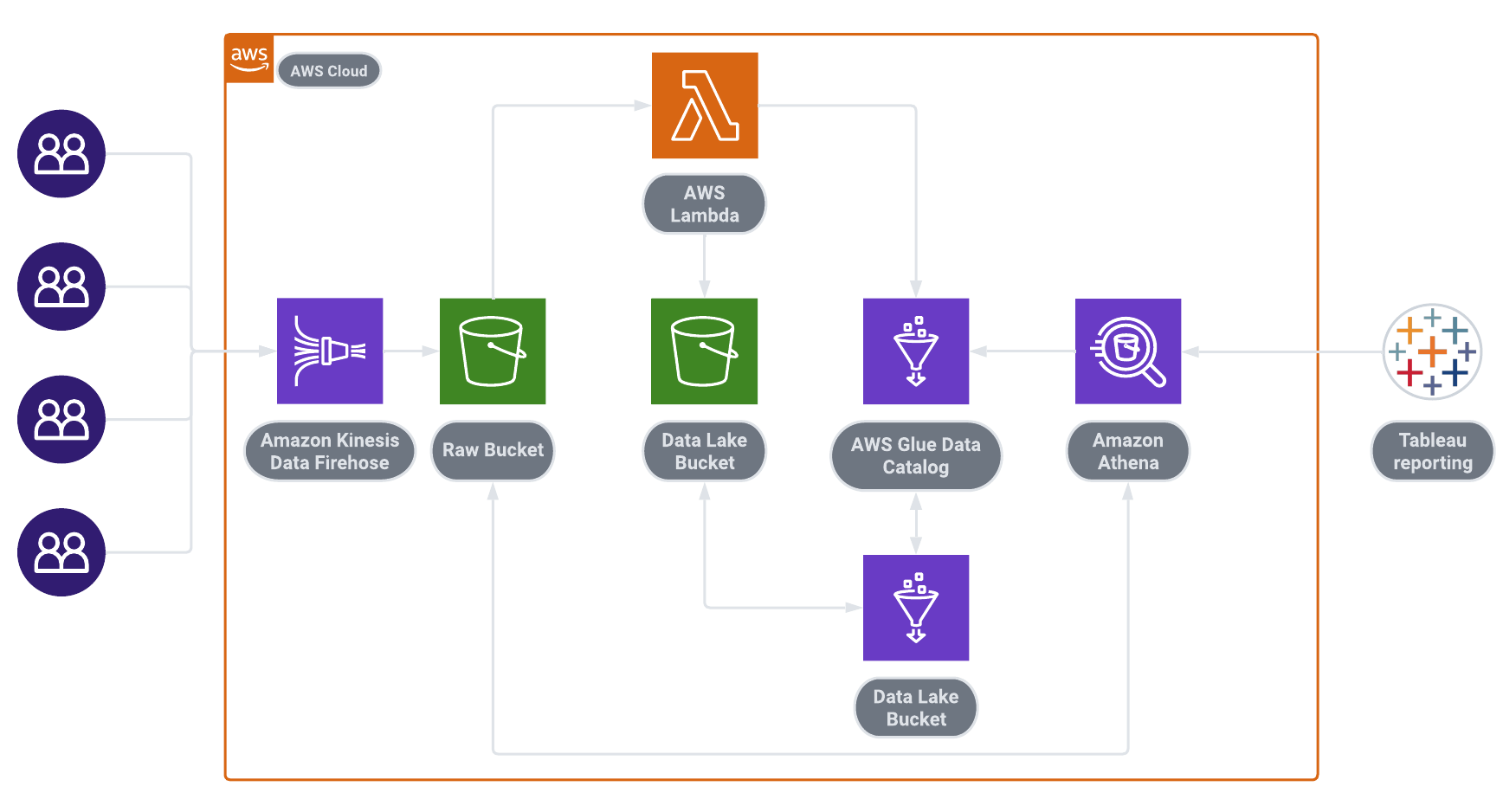
The solution’s operational overhead was further emphasized by the ongoing maintenance of its various configuration components as well as the need for extensive monitoring and ongoing optimization of its crawlers. This led to having a resource on the Behaviour team being affected full-time to the maintenance of this pipeline as every change in analytics requirements necessitated active data engineering efforts to make its way into the pipeline, which would in turn lead to an undesirable fluctuation in data quality.
The Player Insights team came to the conclusion that the right path forward for them was to migrate their current stack to Snowflake, which they had identified as an ideal fit for their functional requirements. The upcoming release of new games meant that they needed to complete the migration as soon as possible. Their day-to-day being filled with Production requests, however, left them unable to tackle such a significant endeavor without external help.
They needed to reduce their pipeline’s engineering overhead, enhance their data quality and start making use of the bleeding edge technology offered by Snowflake without spending months iterating experimentally to develop those features internally.
The challenge
Behaviour operates a 150TB data lake that sees approximately 300GB of data movement on any specific day.
Their existing data pipeline was a custom solution built on top of Amazon Web Services (AWS) services, ingesting files through Kinesis Firehose, storing them in S3 and making them available for querying via Athena. While this solution had its advantages, it was difficult to maintain and lacked some core functionalities to meet the emerging requirements of the Player Insights team.
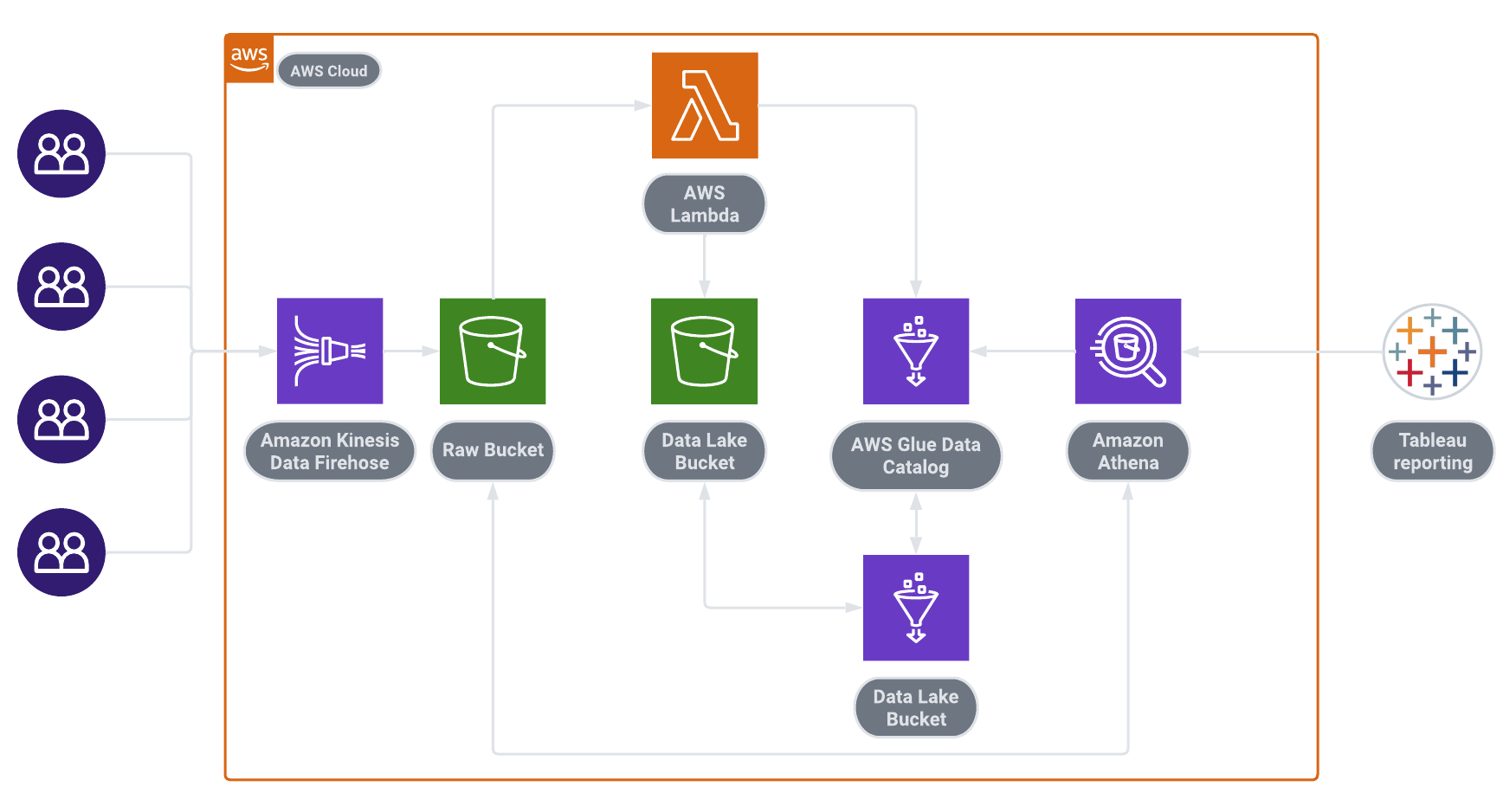
The solution’s operational overhead was further emphasized by the ongoing maintenance of its various configuration components as well as the need for extensive monitoring and ongoing optimization of its crawlers. This led to having a resource on the Behaviour team being affected full-time to the maintenance of this pipeline as every change in analytics requirements necessitated active data engineering efforts to make its way into the pipeline, which would in turn lead to an undesirable fluctuation in data quality.
The Player Insights team came to the conclusion that the right path forward for them was to migrate their current stack to Snowflake, which they had identified as an ideal fit for their functional requirements. The upcoming release of new games meant that they needed to complete the migration as soon as possible. Their day-to-day being filled with Production requests, however, left them unable to tackle such a significant endeavor without external help.
They needed to reduce their pipeline’s engineering overhead, enhance their data quality and start making use of the bleeding edge technology offered by Snowflake without spending months iterating experimentally to develop those features internally.
The solution
Infostrux was brought in to help Behaviour’s internal team to deliver on this project quickly and with a minimal amount of risk. Infostrux’s expertise on the Snowflake Data Cloud combined with Behaviour’s internal knowledge allowed for Infostrux’s DataPod delivery model, where Infostrux and their customer’s team members operate as a joint project team led by Infostrux, to be efficient both in terms of knowledge transfer and delivery.
The project scope was as follows:
- Migrate historical data from AWS to Snowflake
- Design and build a fully automated pipeline between AWS and Snowflake for new data to flow automatically
- Define new data models with Behaviour’s internal team
- Migrate existing queries, functions and views to Snowflake and the new target data architecture
The team started by quickly defining and implementing the necessary configuration to properly integrate Snowflake with Behaviour’s AWS-based infrastructure, leveraging Infostrux’s accelerators to avoid wasting time during the setup phase and sidestepping common configuration pitfalls.
With the Snowflake and AWS environments integrated, the next objective was to put in place a live data pipeline that would allow data to flow between AWS and Snowflake. The criticality and volume of Behaviour’s data meant that the chosen design and implementation strategy needed to optimize both for data resiliency and cost efficiency.
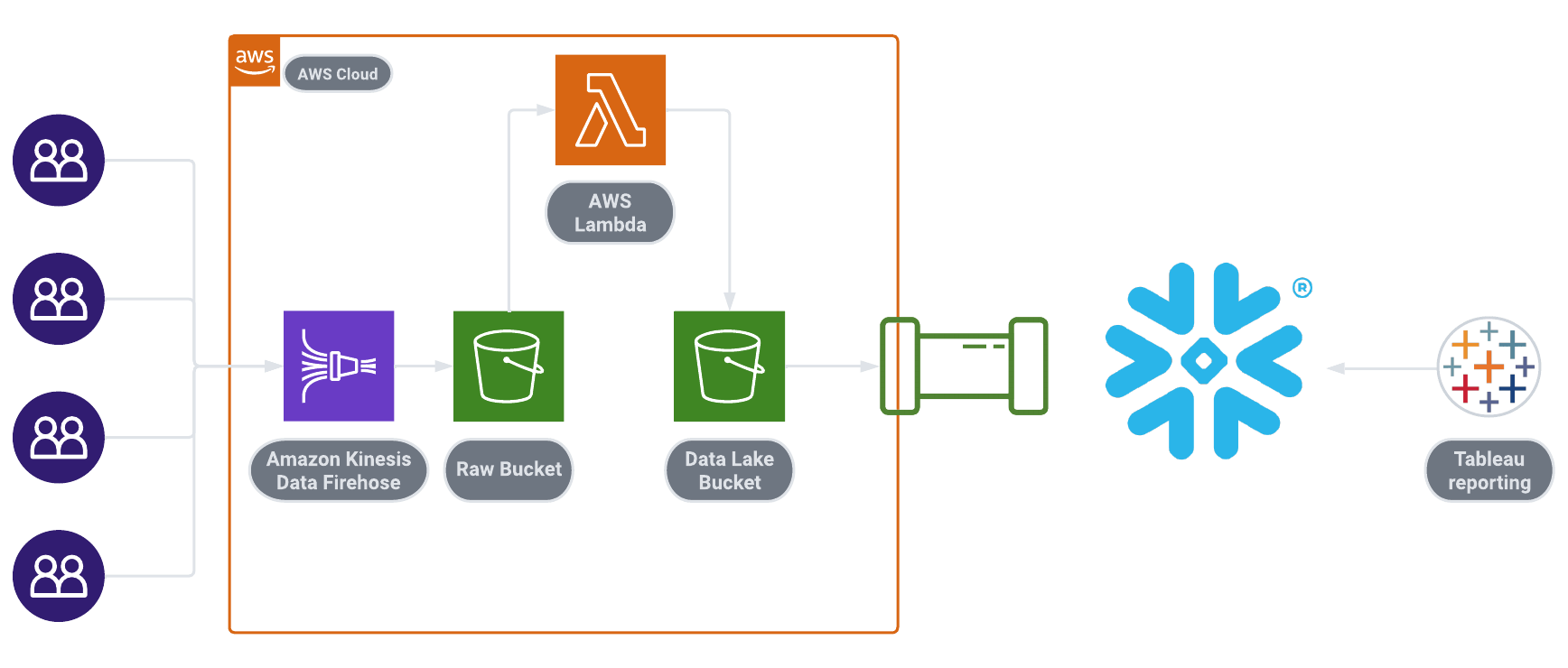
From a design perspective, Behaviour’s existing data extraction components leveraging Kinesis Firehose, Lambda and S3 were deemed viable and cost-effective, but Glue crawlers were deprecated in favor of using Snowpipe to load data into Snowflake, where dbt would then be used to transform the data according to the chosen models. Snowpipe’s ease of use and performance played a significant part in reducing the overall solution’s operational overhead.
The solution was implemented in parallel with the existing data pipeline’s transformation components, leveraging the same extraction infrastructure to ensure that any risk of data loss that could occur in the context of a migration would be alleviated. Additionally, leveraging the same data extraction components allowed for a highly reliable data quality validation strategy where the data outputted by each concurrent data pipeline could be directly compared, and discrepancies immediately identified and addressed.
Once the new pipeline’s functionality was validated, the DataPod worked on migrating the large set of queries, views & functions developed by Behaviour in the existing solution. Infostrux and Behaviour’s teams worked hand in hand to rewrite and prepare all the queries necessary to enable their business-critical metrics & dashboards within the new data platform, as well as optimizing the execution strategy of the underlying dbt transformation layer.
The final outcome of the project was a solution with a significantly reduced operational overhead, a higher level of resiliency and the new focus on Snowflake yielded more functionality out of the box as well as a reduced yearly operation and infrastructure cost.
The solution
Infostrux was brought in to help Behaviour’s internal team to deliver on this project quickly and with a minimal amount of risk. Infostrux’s expertise on the Snowflake Data Cloud combined with Behaviour’s internal knowledge allowed for Infostrux’s DataPod delivery model, where Infostrux and their customer’s team members operate as a joint project team led by Infostrux, to be efficient both in terms of knowledge transfer and delivery.
The project scope was as follows:
- Migrate historical data from AWS to Snowflake
- Design and build a fully automated pipeline between AWS and Snowflake for new data to flow automatically
- Define new data models with Behaviour’s internal team
- Migrate existing queries, functions and views to Snowflake and the new target data architecture
The team started by quickly defining and implementing the necessary configuration to properly integrate Snowflake with Behaviour’s AWS-based infrastructure, leveraging Infostrux’s accelerators to avoid wasting time during the setup phase and sidestepping common configuration pitfalls.
With the Snowflake and AWS environments integrated, the next objective was to put in place a live data pipeline that would allow data to flow between AWS and Snowflake. The criticality and volume of Behaviour’s data meant that the chosen design and implementation strategy needed to optimize both for data resiliency and cost efficiency.
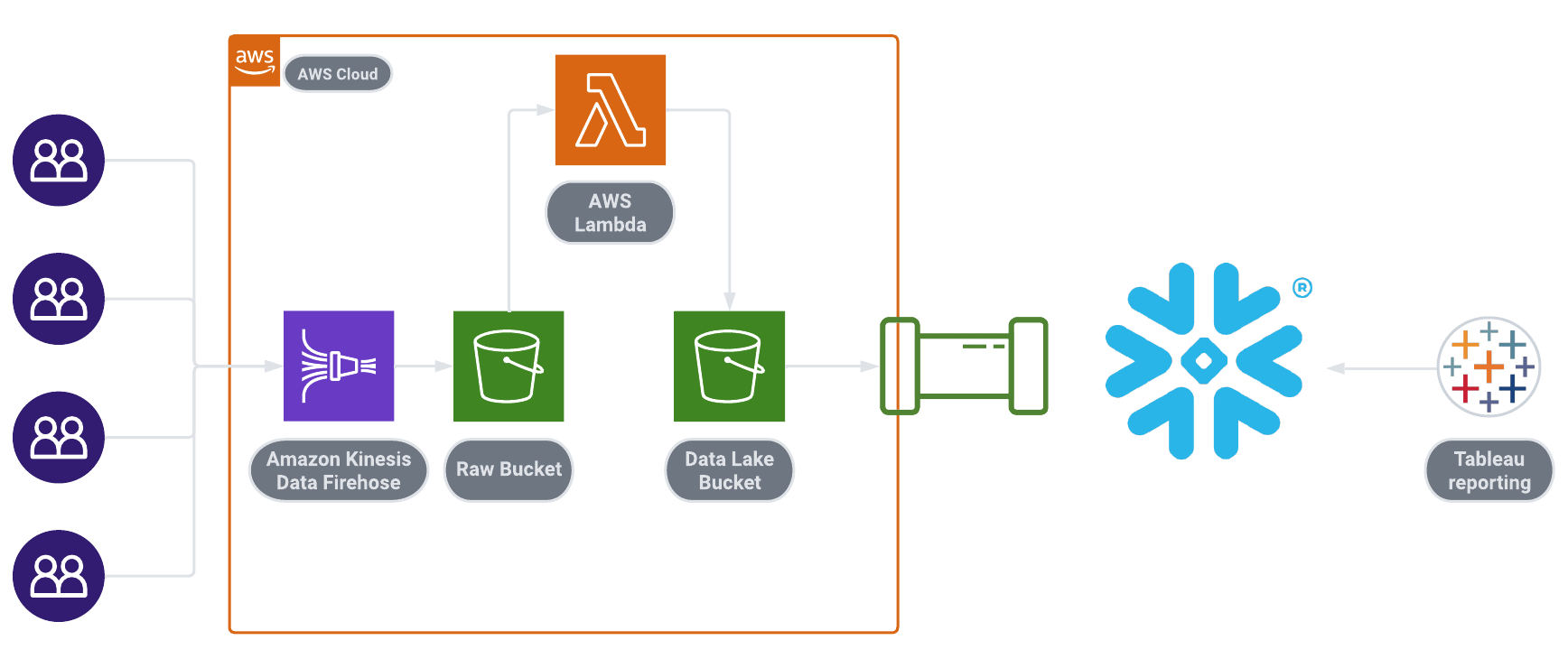
From a design perspective, Behaviour’s existing data extraction components leveraging Kinesis Firehose, Lambda and S3 were deemed viable and cost-effective, but Glue crawlers were deprecated in favor of using Snowpipe to load data into Snowflake, where dbt would then be used to transform the data according to the chosen models. Snowpipe’s ease of use and performance played a significant part in reducing the overall solution’s operational overhead.
The solution was implemented in parallel with the existing data pipeline’s transformation components, leveraging the same extraction infrastructure to ensure that any risk of data loss that could occur in the context of a migration would be alleviated. Additionally, leveraging the same data extraction components allowed for a highly reliable data quality validation strategy where the data outputted by each concurrent data pipeline could be directly compared, and discrepancies immediately identified and addressed.
Once the new pipeline’s functionality was validated, the DataPod worked on migrating the large set of queries, views & functions developed by Behaviour in the existing solution. Infostrux and Behaviour’s teams worked hand in hand to rewrite and prepare all the queries necessary to enable their business-critical metrics & dashboards within the new data platform, as well as optimizing the execution strategy of the underlying dbt transformation layer.
The final outcome of the project was a solution with a significantly reduced operational overhead, a higher level of resiliency and the new focus on Snowflake yielded more functionality out of the box as well as a reduced yearly operation and infrastructure cost.