Structured Data vs. Unstructured Data
Before diving into the difference between a data lake and a data warehouse, it is helpful to understand structured vs. unstructured data.
Structured data is generally maintained in a neat, predictable, orderly format, such as tables or rows and columns in a spreadsheet. This could include customer names, dates, addresses, order history, product information, etc.
Unstructured or semi-structured data refers to data that doesn't conform to traditional structured data standards but contains tags or other types of markup that identify individual, distinct entities within the data, e.g., XML [Extensible Markup Language], web data stored as JavaScript Object Notation [JSON] files, CSV [comma-separated value] files, tab Delimited text files, and so on.
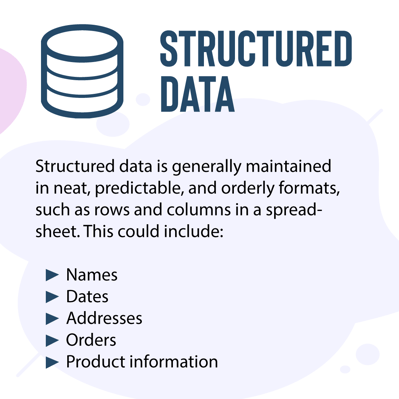
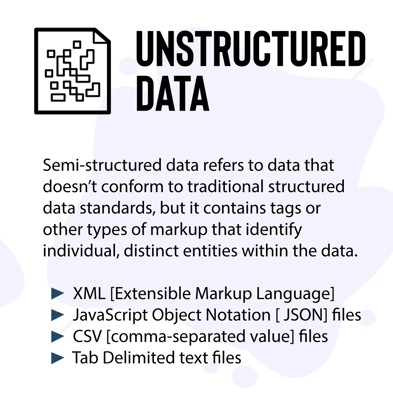
Data Lake vs. Data Warehouse: What is the Difference?
As their names imply, a lake is much larger than a warehouse, so that is a giveaway about their respective storage capacities.
When you think of a warehouse, you likely picture rows upon rows of neatly stacked inventory labeled, categorized, cataloged, and accessible. This analogy holds for a data warehouse.
Traditional data warehouses use an Extract Transform Load (ETL) process, where data is first extracted from the original data sources (such as a CRM, ERP, etc.) and mapped to tables in the data warehouse. Next, it undergoes a transformation stage, where it is put into a structured format. This makes the data consistent, comprehensible, and ready for reporting and BI analysis.
On the other hand, a lake is like a large soup of free-flowing elements in their natural (or “raw") form. The "rawness" of these elements doesn't necessarily mean no structure or organization. When there is no structure or the structure devolves into chaos over time due to a lack of design and planning, your data lake could become a data swamp. This is where proper data quality and data governance measures come into play.
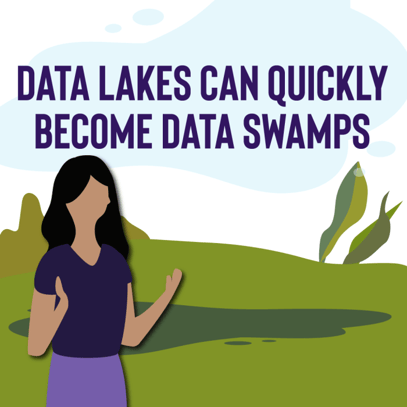
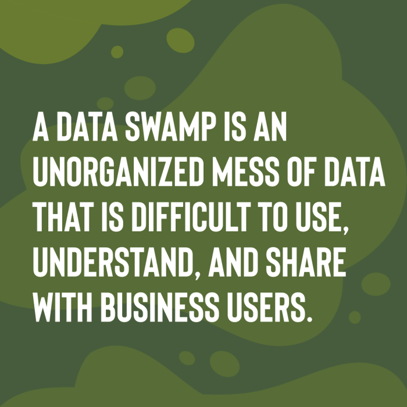
A data lake is a repository for structured and unstructured data in its original form and format. This data is unprocessed and does not yet have a specific purpose, but it may be processed later for future analysis. Since it is unstructured, it typically caters to a more technical user, such as a data scientist or a developer. As mentioned, the storage capacity of a data lake is much higher than a data warehouse.
A data warehouse is a repository for structured data from many applications and sources. The data is cleaned and organized for specific business purposes and ready for BI tool analysis.
You may have also heard the term. Again, the name gives us a clue to its size. It is a smaller version of a data warehouse that stores a partitioned segment, or subset, of data from a business unit such as sales, marketing, finance, etc.
Benefits of Each Type of Storage
Data Lake – There are benefits to having your data in its raw form inside a data lake. One such benefit is accessing and analyzing your data quickly and for any purpose. Since a data lake stores data in its original format, it can be immediately accessible for analysis. Data within a data lake is never deleted, which allows the data to be retrieved for analysis, stored for later use, or shared with others.
Data Warehouse – storing processed data inside a data warehouse has three main benefits.
- Data warehouses have been around for a long time and have a mature security posture.
- Processed data is much easier and more accessible, particularly for less technical users.
- Data warehouse only stores processed data that will be used to answer specific business questions (e.g., what was our gross margin last quarter? How many units did we sell YoY). When you’re just paying for what you need, this means big savings, especially over long periods.
Data Mart – As we saw some cost-saving benefits with data warehouses, data marts are another cost-effective way to gain actionable insights quickly. Since data marts store a partitioned segment, or subset, of data from a business, this can accelerate processes by allowing less technical business users to access relevant information to their particular business unit (i.e., sales, marketing, finance, etc.).
The Role of Data Engineers with Data Lakes and Data Warehouses
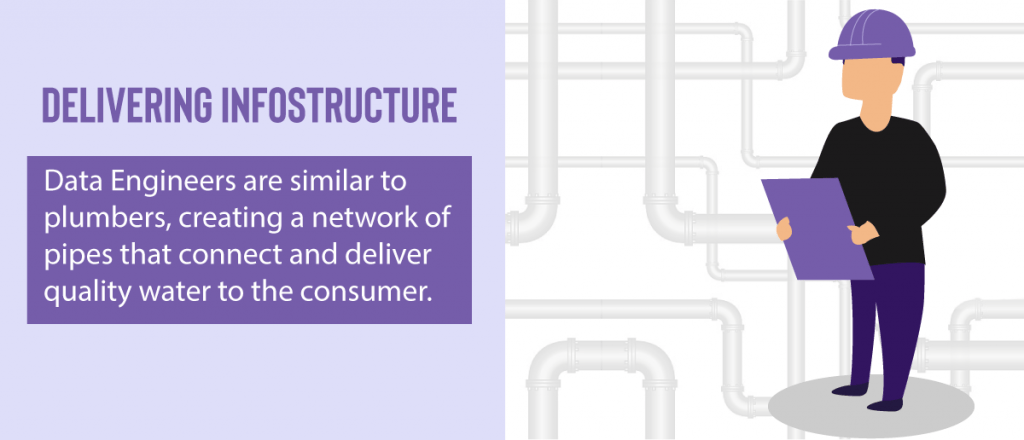
The pipes in which data engineers lay help the incoming data conform to a predefined structure. This enables business units to use business intelligence tools to ask specific business questions and glean insights from their data. Any data that is not needed immediately will not be included in the data warehouse. This is done to improve performance and reduce storage space, which is costly at scale.
However, as data sources increase and data pipelines become more complex, a business’s data storage process must evolve. Data warehouses may be insufficient, and managing all that data becomes increasingly difficult for many companies to handle independently. Expertise is expensive and hard to find. Instead, many companies prioritize the workload of a data scientist who may not have the specialized knowledge and experience to take on such a task.
At Infostrux, we offer data engineering as a service. We help data-driven organizations get the most out of their data.
Snowflake Data Warehouse and Data Lake
Snowflake Cloud Data Platform brings all your structured and semi-structured data together in a single platform, delivering the best storage and analytical attributes of a data lake and a data warehouse.
Snowflake offers unlimited scale, speed, simplicity, cost-effectiveness, and built-in security and governance.
Data lakes running on Snowflake Data Cloud can easily be created and scaled, removing storage limitations. Instead of exorbitant CapEX costs of on-premises data systems, Snowflake has an affordable OpEx, usage-based pricing model. This is cost-effective since it is difficult to predict how much or how little storage you may need. Snowflake's fully scalable, on-demand, pay-for-what-you-need data platform can save companies money.
Snowflake is faster, easier to use, and more flexible than traditional data platforms. These (among other reasons) is why we work exclusively with the Snowflake data cloud platform.
Read: Why We Choose Snowflake
Which one is right for you?
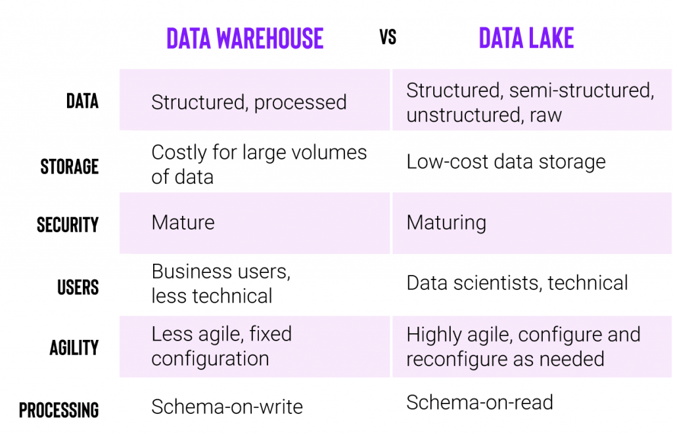